
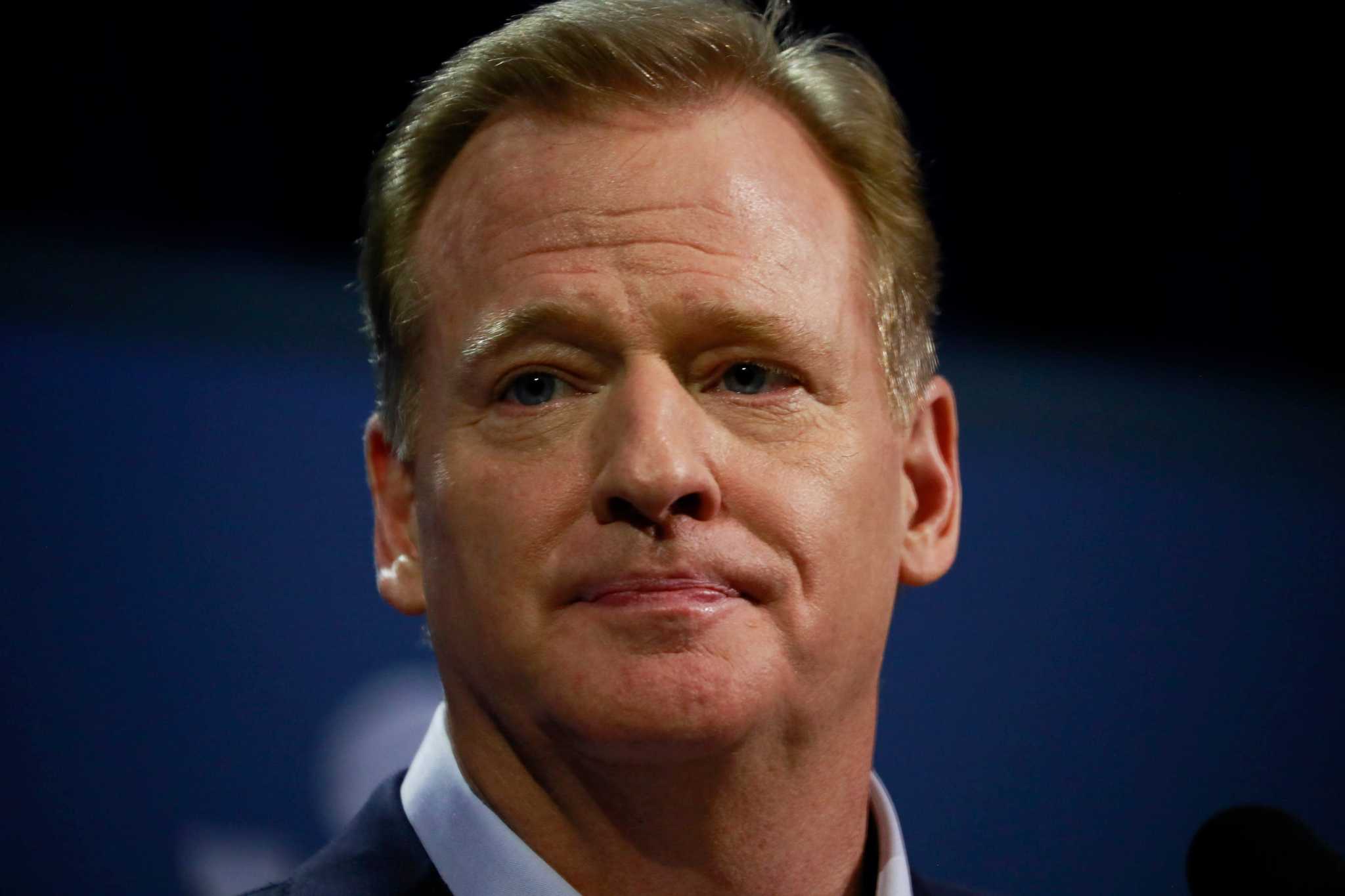
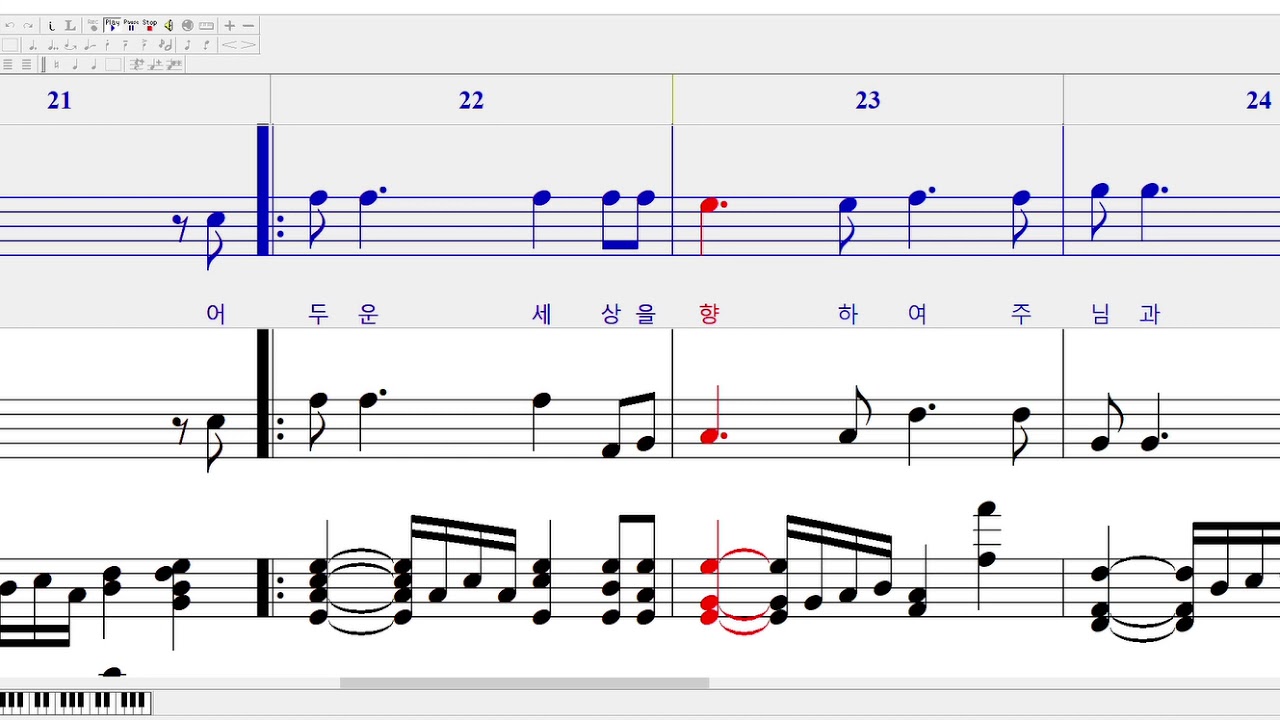
Jagannatha, A.N., Yu, H.: Bidirectional RNN for medical event detection in electronic health records. Guo, D., Duan, G., Yu, Y., Li, Y., Wu, F.X., Li, M.: A disease inference method based on symptom extraction and bidirectional long short term memory networks. Gardner, R.L., et al.: Physician stress and burnout: the impact of health information technology.
#DEFINE NOTEWORTHY SOFTWARE#
In: Workshop for NLP Open Source Software (NLP-OSS) (2018) Gardner, M., et al.: AllenNLP: a deep semantic natural language processing platform. In: International Workshop on Semantic Evaluation (SemEval) (2016) joint inference for clinical temporal information extraction. 24, 596–606 (2017)įries, J.: Brundlefly at SemEval-2016 task 12: recurrent neural networks vs. IEEE (2017)ĭernoncourt, F., Lee, J.Y., Uzuner, O., Szolovits, P.: De-identification of patient notes with recurrent neural networks. In: IEEE International Conference on Bioinformatics and Biomedicine (BIBM). SIAM (2016)ĭatla, V., et al.: Automated clinical diagnosis: the role of content in various sections of a clinical document. In: SIAM International Conference on Data Mining (SDM). American Medical Informatics Association (2001)Ĭheng, Y., Wang, F., Zhang, P., Hu, J.: Risk prediction with electronic health records: a deep learning approach. In: North American Association for Computational Linguistics - Human Language Technologies (NAACL-HLT) (2019)Īronson, A.R.: Effective mapping of biomedical text to the UMLs metathesaurus: the MetaMap program. We find that by first filtering for (predicted) noteworthy utterances, we can significantly boost predictive performance for recognizing both diagnoses and RoS abnormalities.Īlsentzer, E., et al.: Publicly available clinical BERT embeddings. To address this challenge, we extract noteworthy utterances-parts of the conversation likely to be cited as evidence supporting some summary sentence. One methodological challenge is that the conversations are long (around 1500 words) making it difficult for modern deep-learning models to use them as input. We focus on the tasks of recognizing relevant diagnoses and abnormalities in the review of organ systems (RoS). In this exploratory study, we describe a new dataset consisting of conversation transcripts, post-visit summaries, corresponding supporting evidence (in the transcript), and structured labels. In this paper, we explore the possibility of leveraging this data to extract structured information that might assist physicians with post-visit documentation in electronic health records, potentially lightening the clerical burden. Despite concerted efforts to mine various modalities of medical data, the conversations between physicians and patients at the time of care remain an untapped resource of insights.
